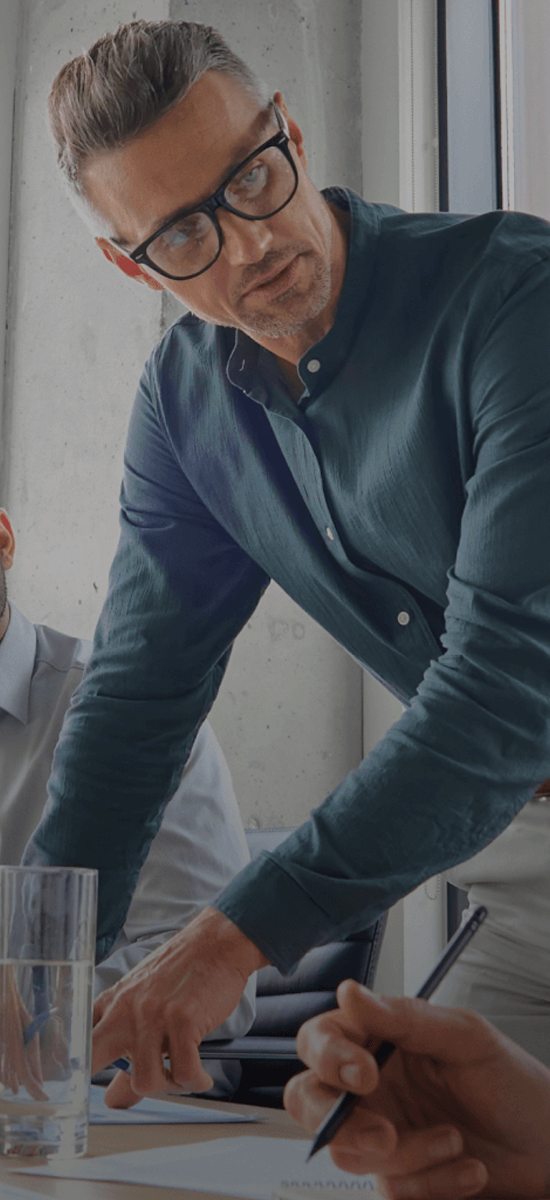
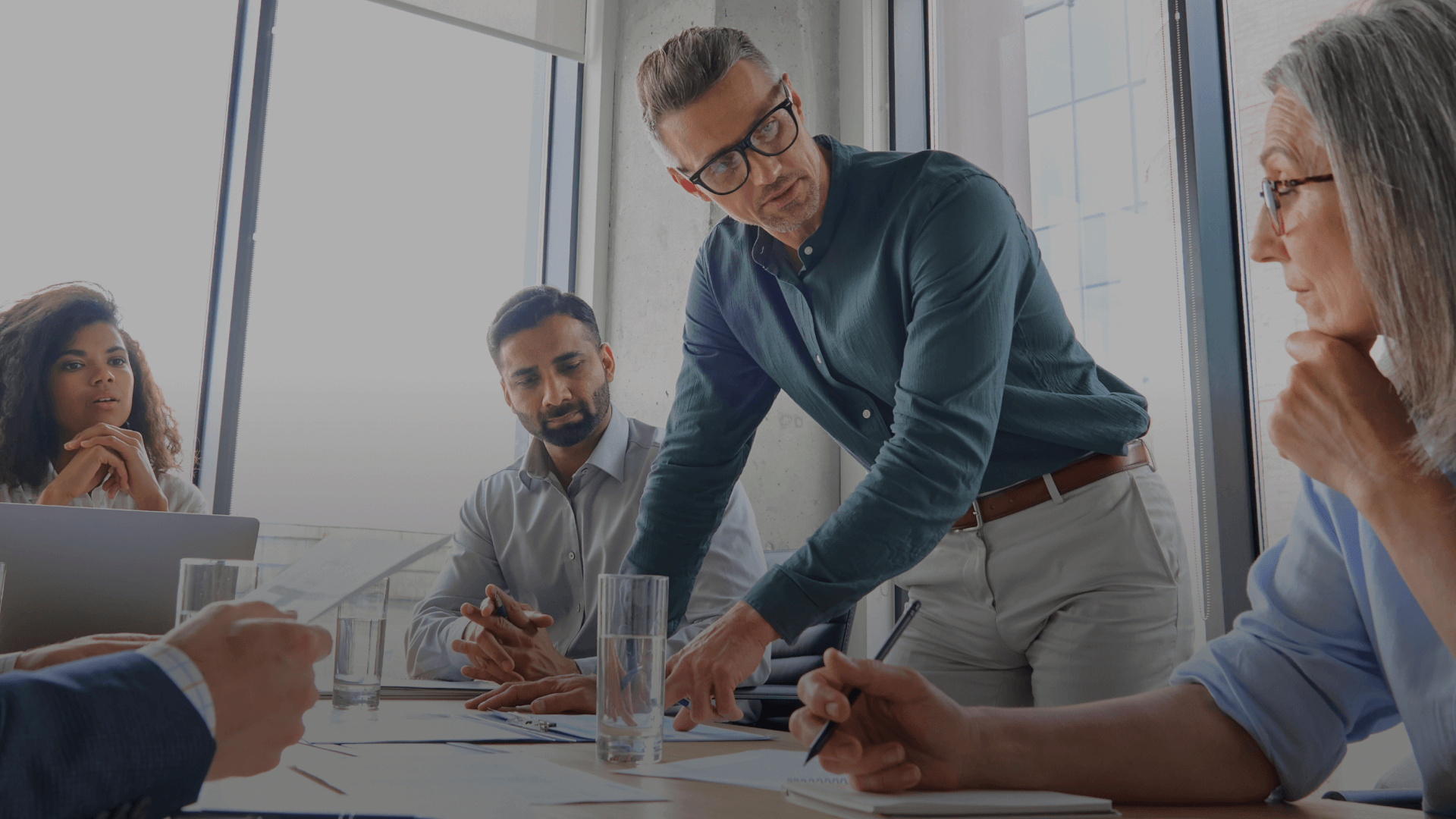
Private Markets Data
Overcoming the private market data challenge.
Dennis Case
Independent Insurance Business Consultant
In requests for proposals for SimCorp’s Alternative Investment Manager, the ability to benchmark private asset funds comes up regularly. To quote: ‘Potential ability to choose a benchmark: Preqin, Cambridge Associates, PitchBook, etc.’
Typical client requests include for example, ‘Can the solution support the ability to upload data (universe/peer group) from different data providers (e.g. Preqin, PitchBook) for private assets?’ and we want ‘also to benchmark the portfolio performance against data from Burgiss’.
But why is this so important for clients? What are the challenges here? And how is SimCorp addressing this?
Importance
Data is the lifeblood of the investment industry. However, players in private markets, regardless of whether they are fund management firms or the so-called limited partners (LPs) that invest in such funds, are reluctant to disclose data on their holdings. Fund management firms – the so-called general partners (GPs) – gain no commercial advantage from transparency and even have a strong motivation to keep as much information confidential as possible. In the fact, the raison d’être of private markets is to legitimately exploit opportunities associated with in-transparency – private equity is called ‘private’ for a reason.
Databases on the private market are created and maintained by mainly four well-established commercial firms: Burgiss, Cambridge Associates (CA), PitchBook, and Preqin. These data providers operate in a difficult market and actually follow different business models to source the data that they can sell on to their subscribers. This has a significant impact on what universe they cover, in which level of detail, and the quality of their data.
Preqin and Pitchbook obtain most of their data from public investors under the Freedom of Information Act (FOIA). FOIA and comparable laws outside the US require LPs to disclose certain information. In addition, both providers make direct requests to LPs as well as GPs for submission. Confidentiality is less of an issue for data of public LPs and therefore in Preqin and Pitchbook individual funds can be identified. On the other hand, fund data harvested from FOIA disclosures or being provided voluntarily tends not to be checked in detail and only covers a sub-space of private markets that is not necessarily representative. PitchBook focuses on venture capital and private equity deals, and therefore fund managers use PitchBook as a tool when conducting due diligence or research. Their data includes LP commitments, cash flows, follow-on financings, exits, benchmarks, and IRR returns data.
As their core business, Burgiss and Cambridge Associates provide fund administration services and thus can provide timely data, with verified accuracy, and arguably less subject to sample biases. Burgiss sources data exclusively from LPs, whereas Cambridge Associates utilizes financial statements provided by the fund managers for their LPs. However, for confidentiality reasons their LP clients only allow fund cash-flows to be made available in an aggregated format. Without cash-flow data for individual funds, there are limits to certain analyses, for instance, those related to cash-flow forecasting.
Data are also expected to be error-prone, as, in fact, relatively few parties work with the data, funds, and portfolio companies are usually not accessible for investment purposes anyway and therefore there is only a limited degree of peer review as a check and balance. Finally, data can be stale, as they are often only updated after significant events. Moreover, reporting of financial statements can be delayed, often significantly. As some databases also cover ‘funds of funds’, their data could result in double counting. It is a general characteristic of an ever-changing alternative market space that innovations and emerging market spaces are initially not recognized as such and wrongly categorized or are simply ignored entirely. As a consequence, all databases will cover high-quality as well as low-quality data.
Gaps in data
Private equity data made available by these providers have significant gaps, as many market participants are not even known. Not all firms contribute data and under-performing fund managers often withhold negative information and drop out. Therefore, it is difficult to assess how complete a picture of the market is provided.
All four providers have similar sample sizes for North American buyout funds but for venture funds, in this region, this varies substantially. Regarding venture funds outside North America, the coverage varies, sometimes dramatically, by the provider. Notably, Cambridge Associates is said to have a larger international sample and better coverage of small funds. In line with the historical development of the private equity markets, notably for the ‘rest of the world’ (Europe, Asia, emerging markets) data are patchy.
For analysts and researchers, the resulting difficulty of obtaining high-quality data creates problems: what conclusions can they draw from outdated and incomplete information and how much confidence can decision-makers put into their findings? No data set may exist on the entire universe of private equity funds, but to some degree, the industry has learned to live with this situation. To paraphrase the late Donald Rumsfeld, as an investor in private assets you need to work with the data you have, not the data you might want or wish to have at a later time.
Interoperability
As the private equity industry becomes more global, globally operating investors, for instance, sovereign wealth funds, require data from multiple countries and often use several data providers in parallel. However (and unsurprisingly), classification schemes are not standardized across different private market data providers. For the various private market databases, the classification schemes differ, often significantly. Examples are how regions and investment styles are labeled, and the label’s granularity, i.e., level of detail.
From a practical perspective, the lack of any uniform classification scheme across the data providers (such as the International Securities Identification Number (ISIN) that uniquely identifies a security) creates a significant barrier to interoperability.
Not having any uniform classification scheme in place also creates problems for clients that are faced with similar incompatibilities regarding how their fund holdings are classified within their own systems. In fact, during SimCorp’s 2022 IUCM a client asked the question ‘how do you match the data from the private market data providers with internal data?’, bemoaning the high workload this regularly caused the client when reporting on comparisons between the assets under management and their peer-groups.
The SimCorp solution
SimCorp’s Alternative Investment Manager now supports a flexible interface to be able to integrate all relevant private market data providers, with Preqin and Cambridge Associates benchmarks already available and other providers expected to be added soon. These data will not just be used for benchmarking but also as input to risk modelling.
To facilitate the inter-operability among different private market data sources, the critical step is to implement a method for mapping between various classification schemes. To integrate data from the different data sources that are organised along broadly comparable lines but have many idiosyncrasies, a single classification scheme is – as explained above – not enough. To overcome this obstacle, a mapping dictionary based approach is used to allow a fuzzy search for the data that match the required piece of information best. Obviously, there is a trade-off between the match and the number of samples that can be found. Most of the time, there will be an insufficient number of samples that precisely meet the user’s requirements. In this mapping dictionary rules define the pairwise matches between classifications of various systems. The dictionary provides a ranking according to match, descending from closest to worst match. With this the number of samples can be controlled as restrictions regarding the degree of match are gradually eased. This allows the SimCorp’s Alternative Investment Manager to use incompatible, imprecise, and precise classifications and mix them freely.
This solution helps SimCorp clients to access data across various providers and other sources. This supports an analytical functionality that so far was only available to few large asset managers with deep pools of proprietary private market data.